AI Business Transformation Roadmap: How to succeed in AI adoption
(Из ленты Курсы бизнес анализа, тренинги и обучение бизнес аналитике| ArtofBA)
This article is a compilation of pieces I’ve developed over the last couple of months to assist marketing and sales departments in their efforts to communicate value of the proposed solutions to the potential or existing clients.
Today AI implementation is a necessity to maintain a competitive advantage in the market regardless of industry. It just makes things easier.
However, AI transforming business ain’t happens out of thin air. The business needs to adapt and build itself up towards implementing machine learning applications and making the most out of them.
AI strategy 101 — Understanding that AI / ML is a tool and not a magic wand
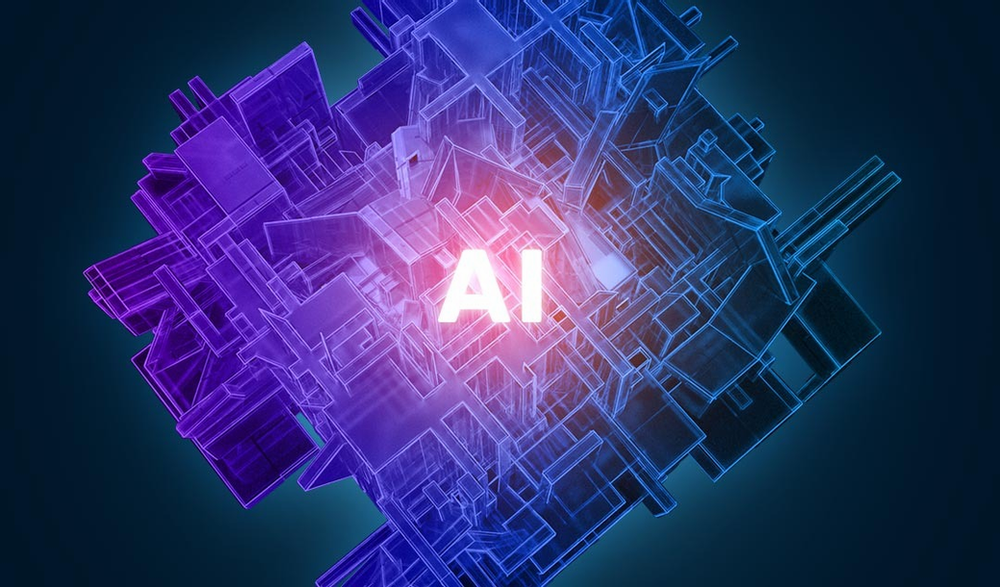
It is as simple as that. The first thing the company should understand about AI implementation in the business process is that it is not a magic wand by any means. It is a solution to particular part of the workflow. That is one of the first steps to implement AI into the business workflow.
Artificial intelligence and machine learning are capable of outstanding results if the rest of the enterprise infrastructure is there to enable it. It cannot pull rabbits out of a hat. As Thomas Davenport put it, “AI will improve products and processes and make decisions better informed.”
The reality is that it is a tool that can help with specific aspects of the business operations — by providing appropriate scope and gaining valuable insights by mining existing data assets and gathering new ones.
As such, the plan for implementing AI in business is shaped by its role in the workflow. No less, no more.
Here are the key AI adoption challenges you to need to address:
- The most common challenge comes from the underestimation of what AI is capable of doing and how the organization can leverage on it. AI can’t point out what decision to make. It can only make the patterns in data more apparent for that.
- On the other hand, there is an underestimation of the company’s capabilities in implementing the required solutions and maintaining its infrastructure.
- AI implementation strategy starts as a long and winding process of experimentation and learning. It takes time (sometimes a lot of time) for AI/ML solution to start generating value within business workflow.
- Due to a variety of options on the market — there is a need to navigate in available AI/ML solutions and understand what fits your needs, whether it is compatible with the rest of the technologies and to what extent you need to adapt the system to implement a particular solution. Because of the limited understanding of impact — it is hard to estimate all pros and cons correctly.
- Traditional performance indicators for AI solutions are Quality of results and Return on investment estimation. The latter is out of the picture until the solution has proven its effectiveness in solving the business need.
- There are numerous regulations regarding data collection, data processing, and data privacy. European Union got GDPR, USA got HIPAA, and local privacy acts, Canada got PIPEDA. The solution needs to stay within the boundaries of relevant regulations.
Understanding the Technology behind AI transformation
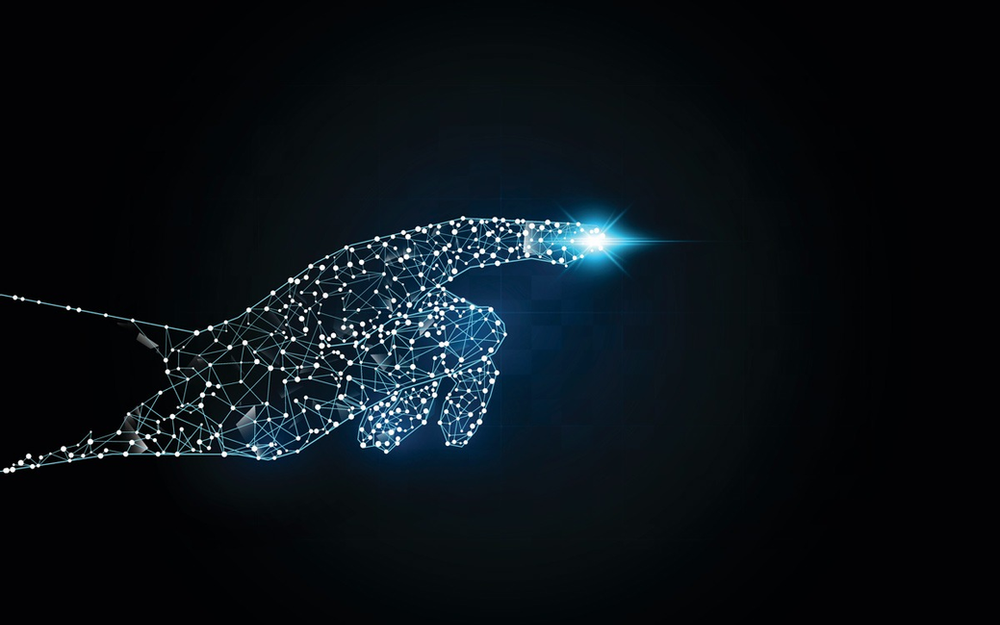
The next step in the AI transformation playbook is exploring viable technologies. The adoption of machine learning artificial intelligence solutions in the business operation relies on the three pillars:
- Data to process and gain valuable insights;
- The infrastructure that processes data and gets valuable insights;
- Human Resource or Talent who processes data via infrastructure and generates value;
The three pillars mentioned above correspond with three main requirements for AI business transformation. As Andrew Ng defines it:
- Understanding of the organization’s AI/ML use cases — what AI can do for the company and how it will affect the business?
- Resources to do AI operations systematically. This aspect includes both technologies to enable AI operation and talent to handle it.
- Strategic direction — the understanding of why the company is doing what it is doing with AI projects. For example, the company is making data analytics solutions, and its goal is to expand the availability of tools and increase the quality of performance.
According to O’Reilly Media survey, one of the reasons why the AI implementation strategy fails is because of:
- Lack of understanding of the business need;
- Failure to implement appropriate technological solutions for business needs;
- Lack of knowledge of what kind of data the organization needs and where to find it. Later on, the issue also includes poor quality of data;
- Shortage of talent and failure to attract one;
Now let’s get back to three pillars of AI transformation.
Infrastructure is the easiest to explain out of all three. In a nutshell, it is a technical solution for a particular task — a combination of hardware and software that makes the whole thing ticking. For example:
- machine-learning frameworks,
- cloud platform for computing operations,
- data storage for datasets and so on.
The design of each infrastructural solution revolves around specific tasks. Let’s look at the most common:
- Data collection — getting data into the system
- Data processing — making sense of data
- Data mining — getting stuff that matters out of data
- Data integration — implementing insights into the business operation
- There’s also data presentation that makes insights comprehensible for civilians via data analytics, data visualization, and the likes.
In the context of the AI implementation strategy, data is tricky. It is the spice, and as you know — spice must flow.
- Data is the lifeblood of the business workflow. The data that the algorithm processes and results it can get out of it define its worth to the business operation.
- The challenge is that relevant data is not up for grabs. There are rules for obtaining data. 1. The company can collect data from its channels; 2. Or it can buy somebody else’s data.
- Its clarity measures the worth of data. How well is it described? How are different data points connected to each other? Usually, data is fragmented and needs to be culled together to present anything of worth. That’s the task of data processing.
There are two long term goals related to data:
- Maintaining the quality of data, i.e., its relevance to the organization and value of insights gained from it.
- Governing data assets, i.e., keeping data safe, controlling its pipeline, maintaining strict data use policies, implementing security, backup protocols, disaster recovery scenarios, and so on.
Speaking of talent or human resources — there is no need to explain what’s their role in this kerfuffle.
But it is important to note that AI talent is not cheap. Sometimes it is better to outsource these experts and combine their efforts with internal business analysis and business intelligence departments. It is a much more cost-effective approach, especially at the early stages of AI transformation by the company.
AI Strategy 101 — Essential components
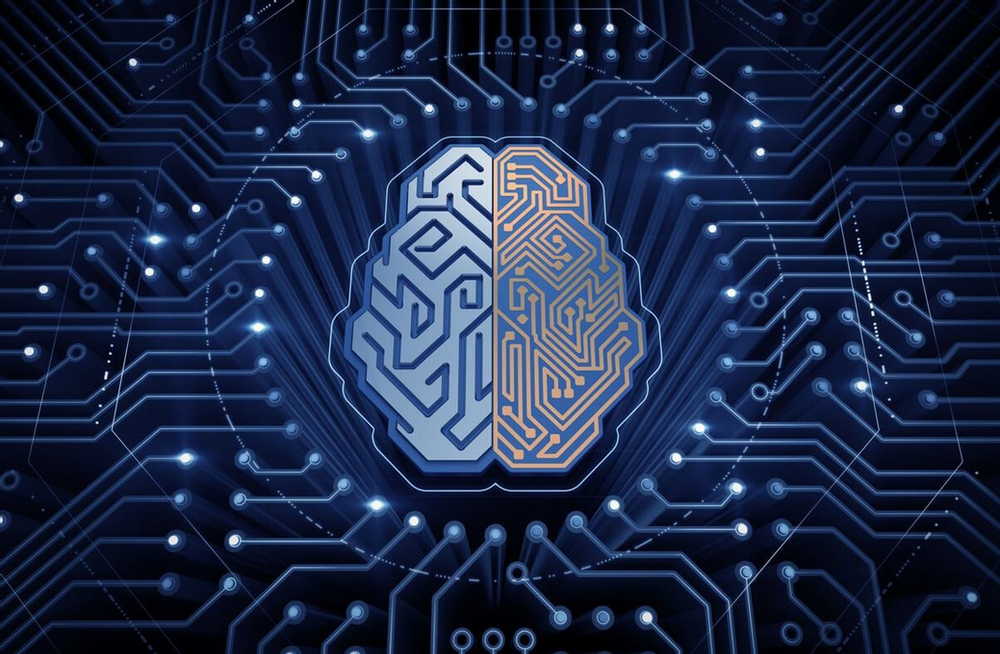
In his “Good Strategy, Bad Strategy,” Richard Rumelt defines AI strategy as a combination of three elements:
A. Diagnosis — this stage is about the assessment of the current state of things. What is going on? What’s good about it? What’s wrong with the way things are going?
B. Guiding policy. This part consists of four parts:
- Foundation — the operational framework of the organization — the way technical infrastructure, enterprise infrastructure are connected by the solution infrastructures.
- Governance — the way functional components of the organization and its technological stack align and operate;
- Culture — the guiding concepts that drive the organization and underline their use of solutions;
- Ethics — boundaries in which the organization is operating.
C. Coherent action, aka decision-making. This stage is all about transformation and change. Coherent action includes such things as:
- resource allocation,
- Solution implementation,
- buy/build decisions,
- process orchestration — both solution-centric and workflow-related
- talent development/hiring/retention — to adequately handle a solution and realize AI strategy
- culture and change management — to maintain a productive environment within the organization.
These components enable overcoming obstacles and seizing opportunities. That’s the foundation for the plan for implementing AI in business.
It seems clear, right? Well, a lot of it usually goes wrong. Here’s how:
- Mistaking goals for strategy
- Stating Vague Objectives — no plan how to get to the goal, no understanding of capabilities and resources
- Lack of Coordinated Actions — no unifying vision, everyone is not on the same page. It ends up being a jumbled mess.
- Inability to Choose and Prioritize— what to do first? What to do next? Which steps are essential? Do we need that much stuff at this point? The list goes on.
I can go into more detail regarding the role of the company’s culture in the AI transformation roadmap.
Here’s what matters the most:
- AI business transformation requires cooperation between different groups for the common goals. More often than not, this includes relegation of building machine learning models for specific AI tasks to third parties. This aspect means there needs to be clarity regarding who is doing what and why and in which order.
- In one way or another, AI adoption disrupts the existing workflow. The company can soften the impact by gradually adapting and expanding the workflow.
- AI implementation requires new data governance policies. This policy includes the following things: the way data is available for the business operation, which elements of it must be reiterated (mostly notably anonymization or pseudonymization of personally identifiable user data) to comply with regulations, how to keep it safe and sound, and so on.
The challenging part of AI transformation is to understand how to fit an AI/ML solution into the specific business workflow without disrupting and, at the same time bringing the whole thing to a new level.
This process requires an understanding of internal and external factors. For example:
- The external factor — Market situation in a particular industry segment.
- Internal factor — The financial capacities of the organization.
- Internal/External factor — company’s technological expertise in a specific field.
In business terms, the ai business transformation more or less goes in an equation like this:
- Artificial Intelligence solution consists of data combined with algorithms that perform specific tasks.
- The business problem shapes the tasks.
- The results of the algorithm’s operation present a solution to a business problem.
Here what it means:
- Artificial Intelligence Machine Learning solutions revolve around business outcomes and then reflect on common enterprise functions and solutions.
- For example, a data mining solution is applicable for numerous purposes and not just audience segmentation.
Steps to implement AI — Checklist for AI transformation
And now we’re getting to the How-To extravaganza. Buckle up.
Gartner provides a list of handy questions regarding how to integrate ai into your business you need to answer. Our interpretation expands upon them based on our own experiences.
These questions will help you to understand whether or not you are moving in the right direction:
- Is the technological solution working as it is supposed to work?
- Is the solution affordable for the company?
- Is the solution cost-effective in the present business workflow?
- Is the software and hardware infrastructure in place to enable AI-fueled operation?
- Is the solution compliant with all relevant regulations? (GDPR and the likes)
- Is the value proposition of the solution resonating with the value proposition of the company?
Here’s how the AI transformation playbook looks like:
- There are Technology Use Cases — for example, predictive analytics
- Some technologies enable the use case — like NLP tools, ML frameworks, Data Science approaches, and so on.
- The combination of the two is projected on business applications of the solution — for example, data security or process automation or customer service.
- Each business area is related to a specific enterprise function — marketing, security, customer support, and so on.
- The solution generates specific outcomes for each department. It can be customer retention for sales and marketing. Or customer satisfaction for customer support. Or better decision making for everyone.
In terms of business operation, it goes like this:
- Business strategy binds the focus of AI solution.
- Business AI strategy defines: 1. Use cases that require an AI solution; 2. Data and sources needed to enable AI solution.
- Then comes vendor evaluations for different aspects of the solution: 1. Business — assessing the pros and cons of different cloud vendors; 2. Technology — checking the performance stats of different ML frameworks.
- This process leads to a period of experimentation that proves in practice whether a solution is capable of handling business needs.
- If proven successful — the solution is transitioned to full-fledged production, and the cycle of AI business transformation is complete.
In conclusion
The 2010s was an incredibly hot decade for AI transforming business operations into more efficient things. Due to the rise of cloud adoption, the operational efficiency of AI had risen to previously unimaginable heights. The models became easier to train and maintain, the gathering of the dataset became less of a chore because machine learning algorithms are now actively helping.
But the most important thing was that AI became an option not only for big corporations. It became available for companies of all sizes and operations. Pretty much anyone with enough expertise now can adopt artificial intelligence technologies for their benefit.
Тренинги от «Art of Business Analysis»:
Комлексный ОНЛАЙН тренинг по бизнес-анализу
Комплексный тренинг по бизнес-анализу
Базовые компетенции бизнес-аналитика
Power BI: Создание решения для бизнеса
Источник: AI Business Transformation Roadmap: How to succeed in AI adoption